Harnessing the Future: Insights into AI Search Innovations and Trends
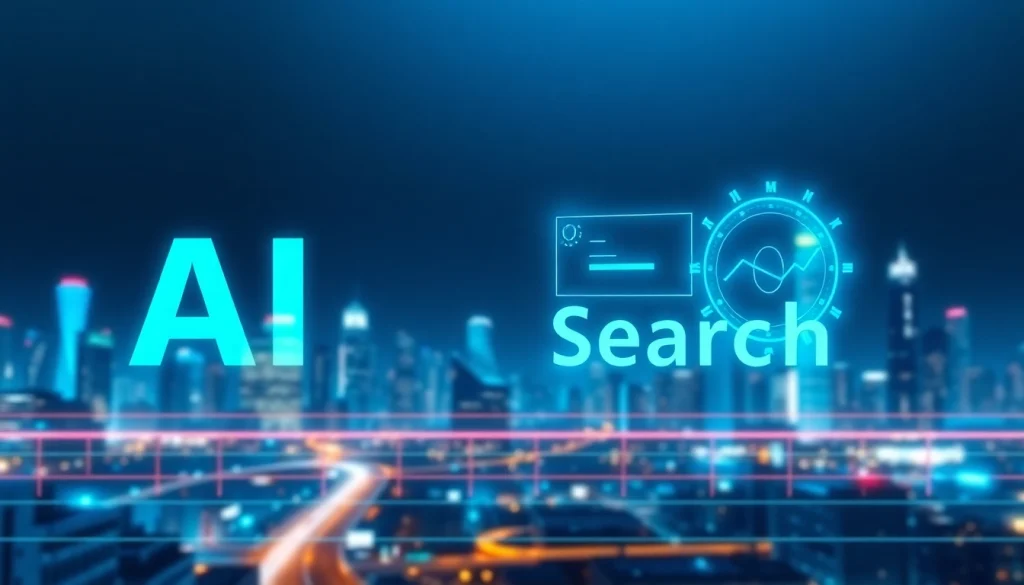
Understanding AI Search Technology
The landscape of information retrieval has been transformed by the advent of AI search technologies. These innovations streamline data access and enhance the accuracy of results, making them indispensable for businesses and users alike. As AI search continues to evolve, understanding its underpinnings and implications becomes increasingly essential.
What is AI Search?
AI search refers to the use of artificial intelligence technologies to enhance traditional search engines. Unlike standard keyword-based searches, AI search utilizes natural language processing (NLP), machine learning algorithms, and semantic understanding to comprehend context, intent, and user preferences. This results in more accurate, relevant, and personalized search outcomes.
Key Technologies Driving AI Search
The evolution of AI search has been propelled by several technologies:
- Natural Language Processing (NLP): Enables machines to parse and understand human language, allowing users to search using conversational queries.
- Machine Learning: Learns from user interactions and feedback, continually refining algorithms to improve search accuracy and relevance.
- Data Mining: Extracts meaningful patterns and insights from vast datasets, enriching search capabilities.
- Semantic Search: Facilitates the understanding of the meaning and context of queries, moving beyond mere keyword matching.
The Evolution of Search Engines with AI
The integration of AI into search engines marks a paradigm shift from traditional keyword-centric models to more intuitive, user-centric systems. Early search engines relied heavily on algorithms based on page ranking and keyword frequency. However, the introduction of AI technologies has enabled search engines to consider user behavior, preferences, and even emotional context, leading to revolutionary improvements in how information is accessed and retrieved.
Benefits of AI Search Systems
Enhanced User Experience
AI search transforms user experience by making the process more intuitive and responsive. By understanding natural language and context, AI systems can return results that resonate with user intent. This reduces the time spent sifting through irrelevant results and enhances overall satisfaction.
Improved Accuracy in Results
Through advanced algorithms and continual learning, AI search engines provide significantly improved accuracy in results. They leverage vast amounts of data to understand and predict user behavior, resulting in highly relevant and precise outcomes, even for complex queries.
Time-Saving Solutions for Businesses
Businesses can harness AI search to streamline operations and enhance productivity. By integrating AI search functionalities, organizations can improve internal knowledge retrieval, automate customer queries, and reduce the time employees spend searching for information. This operational efficiency translates into cost savings and more effective decision-making.
Comparative Analysis of Leading AI Search Engines
Performance Metrics and Features
When evaluating AI search engines, several performance metrics are critical:
- Response Time: How quickly an AI search engine returns results affects usability and engagement.
- Accuracy: The relevance of search results is paramount. Metrics such as precision and recall offer insights into effectiveness.
- User Satisfaction Scores: Gathering user feedback on experience can help gauge the efficacy of AI search enhancements.
User Feedback and Case Studies
Real-world applications of AI search technology have shown diverse results across industries. For instance, in the healthcare sector, providers using AI search engines saw improved patient outcomes by facilitating faster information retrieval for medical professionals. Case studies illustrate the direct correlation between AI search implementation and enhanced operational efficiency across various domains, from retail to education.
Market Positioning of Various Platforms
Major players in the AI search market include Google, Microsoft, and several startups that are rapidly emerging. These platforms often differentiate themselves through unique features and algorithms, catering to specific user needs. For instance, Google has integrated AI into its search algorithms to optimize user experiences with personalized results, while Azure AI Search focuses on enterprise solutions that facilitate knowledge retrieval across organizations.
Best Practices for Implementing AI Search
Integrating AI Search into Existing Systems
To successfully integrate AI search, organizations should begin with a clear understanding of their user needs and existing system architecture. Steps to consider include:
- Assessment: Evaluate the current search system and identify areas for improvement.
- Data Management: Ensure that data quality and accessibility are prioritized to support AI functionalities.
- Training: Provide training for users and administrators to maximize the benefits of the new system.
Common Challenges and Solutions
Implementing AI search is not without challenges, including data privacy concerns and user acceptance. Solutions to these challenges may include:
- Transparency: Maintaining transparency about how AI processes data can build trust with users.
- User Engagement: Actively involving users in the implementation process can help to alleviate concerns and foster acceptance.
- Continuous Improvement: Regularly update and optimize AI algorithms based on user feedback and performance metrics.
Monitoring and Optimizing AI Search Performance
To ensure sustained effectiveness, it’s vital to engage in ongoing monitoring and optimization of AI search performance. This can involve:
- Analytics Tracking: Utilize analytics tools to monitor user interactions and engagement with search results.
- A/B Testing: Conduct experiments to compare different AI search implementations and their outcomes.
- User Feedback Collection: Actively solicit and analyze feedback to identify areas for further enhancement.
The Future Landscape of AI Search
Upcoming Innovations and Research Directions
The future of AI search holds exciting possibilities with advances in technology. Innovations on the horizon may include:
- Voice Search Integration: As voice-activated search becomes more prevalent, AI search capabilities will likely adapt to deliver increasingly accurate voice-based results.
- Visual Search Expansion: With advances in image recognition, visual search is set to enhance user experiences by allowing users to search using images instead of text.
- Hyper-Personalization: Leveraging AI to offer tailored search results based on individual behaviors, preferences, and contextual factors will become more common.
Ethical Considerations in AI Search
As AI search systems continue to evolve, ethical considerations in their development and implementation will be paramount. Key ethical issues include:
- Bias in Algorithms: It’s crucial to recognize and mitigate biases in AI algorithms that may affect search outcomes and perpetuate inequalities.
- Data Privacy: Safeguarding user data and transparency in data usage must remain a priority to foster trust.
- Accountability: The responsibility for how AI search systems are designed and the outcomes they produce must be clearly defined.
Preparing for a Multimodal Search Experience
The rise of multimodal search—combining text, voice, and visuals into a single interface—marks a significant turning point in information retrieval. Businesses and developers must be ready to embrace this shift by:
- Expanding Capabilities: Invest in technologies that enable multimodal inputs and outputs.
- User-Centric Design: Design interfaces that accommodate various forms of user input, making search as seamless and intuitive as possible.
- Continuous Learning: Stay updated with the latest research and innovations in search technology to remain competitive.